
- #TIMENET TIME SERIES CLASSIFICATION MANUAL#
- #TIMENET TIME SERIES CLASSIFICATION SKIN#
- #TIMENET TIME SERIES CLASSIFICATION SERIES#
#TIMENET TIME SERIES CLASSIFICATION SKIN#
With the advancement in technology and rapid increase in computational resources, various machine learning techniques and deep learning models have emerged for the analysis of medical images most especially the skin lesion images.
#TIMENET TIME SERIES CLASSIFICATION MANUAL#
This manual examination of skin lesions in order to detect melanoma can be time-consuming and tedious. Finally, experimental results are presented for the CDnet 2014 dataset.Īnalysis of skin lesion images via visual inspection and manual examination to diagnose skin cancer has always been cumbersome. We then discuss the adequacy of deep neural networks for the task of background subtraction. To do so, we first surveyed the background initialization and background subtraction methods based on deep neural networks concepts, and also deep learned features. In this context, we provide the first review of deep neural network concepts in background subtraction for novices and experts in order to analyze this success and to provide further directions. Furthermore, since the seminal work of Braham and Van Droogenbroeck in 2016, a large number of studies on convolutional neural networks applied to background subtraction have been published, and a continual gain of performance has been achieved. The top background subtraction methods currently used in CDnet 2014 are based on deep neural networks, and have demonstrated a large performance improvement in comparison to conventional unsupervised approaches based on multi-feature or multi-cue strategies. Convolutional neural networks, which are used in deep learning, have been recently and excessively employed for background initialization, foreground detection, and deep learned features. Indeed, the well-known Self-Organizing Background Subtraction (SOBS) method and its variants based on neural networks have long been the leading methods on the large-scale CDnet 2012 dataset during a long time.
#TIMENET TIME SERIES CLASSIFICATION SERIES#
We present the algorithm's performance on the time series archive, where the result shows that using meta-learning algorithms leads to faster convergence with fewer iteration over the non-meta-learning equivalent.Ĭonventional neural networks have been demonstrated to be a powerful framework for background subtraction in video acquired by static cameras.
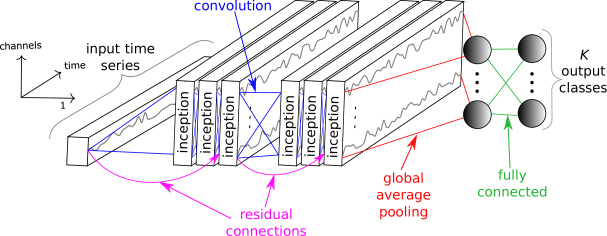
In this work, we aimed to understand the effectiveness of meta-learning algorithms in time series classification task with multivariate time-series datasets. Much work has already been done on meta-learning in various learning environments, including reinforcement learning, regression task, classification task with image, and other datasets, but it is yet to be explored with the time-series domain. It solves the traditional machine learning paradigm problem, where it needed a vast dataset to learn any task to train the model from scratch. Meta-Learning, the ability of learning to learn, helps to train a model to learn very quickly on a variety of learning tasks adapting to any new environment with a minimal number of examples allows us to speed up the performance and training of the model. The qualitative analysis highlights the categories of top citation rate papers by describing them in details. The research field has been broken down into three main categories as different frameworks of deep neural networks, different applications in remote sensing and also in signal processing for time-series classification tasks. The annual number of publications, the productive and collaborative countries, the growth rate of sources, the most occurred keywords and the research collaborations are revealed from the bibliometric analysis within the study period. The paper continues by exploring the publication trends of recent deep learning approaches for time-series classification. Through keywords co-occurrence analysis, a visual network structure of top keywords in time-series classification research has been produced and deep learning has been introduced as the most common topic by additional inquiry of the bibliography. The paper conducts a bibliometric analysis on related publications in time-series classification, adopted from Scopus database between 20.

The quantity of related publications requires a bibliometric study to explore most prominent keywords, countries, sources and research clusters. Among the broad range of time-series classification algorithms, recent studies are considering the impact of deep learning methods on time-series classification tasks. Time-series classification is utilized in a variety of applications leading to the development of many data mining techniques for time-series analysis.
